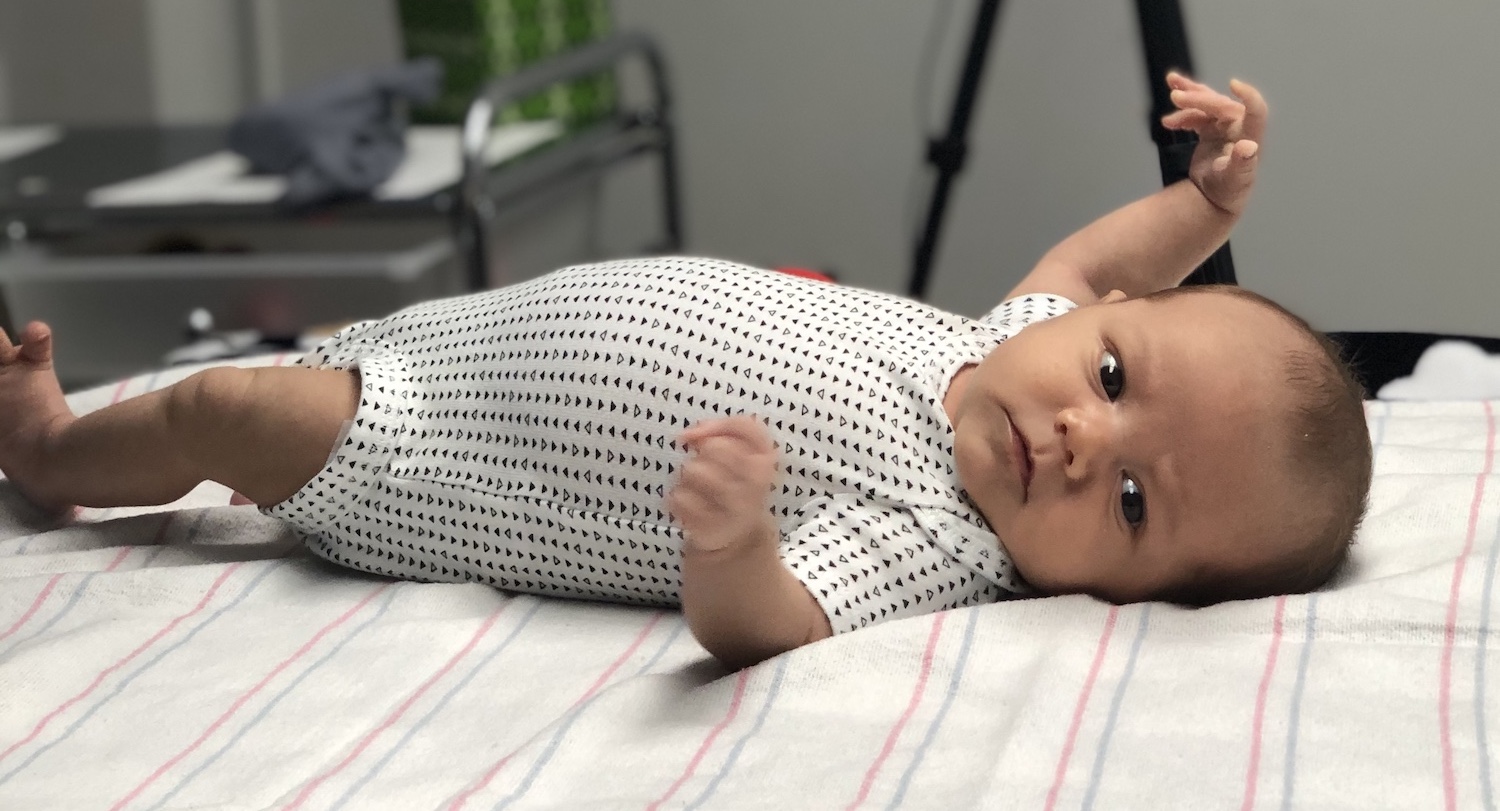
Analyzing large datasets of heart rhythms and brain wave activity with AI and machine learning, a team of University of South Carolina professors is making progress toward better understanding of autism spectrum disorder and identification of ASD diagnostic biomarkers.
Jessica Bradshaw and Caitlin Hudac in the Department of Psychology are collaborating with Christian O’Reilly, a computer science and engineering faculty member, to search for potential patterns and markers of ASD and more.
“I think the punchline for where we’re going together in our collaboration is understanding how we can use all of the information we’re gathering and put it together — and that's where AI and machine learning are so important,” says Hudac, steering director for the Carolina Autism and Neurodevelopment Research Center.
“My data are important for understanding which brain substrates are responding, and Dr. Bradshaw’s data show us trajectories of growth in relevant domains to autism. What really needs to be happening is putting all of these pieces together in one pot to understand each individual.”
Identifying reliable biomarkers of ASD to diagnose the disorder soon after birth as opposed to the standard norm of diagnoses at 3-4 years of age or older using behavioral markers would be a breakthrough, Bradshaw says, because it would allow much earlier therapeutic intervention. The researchers’ work could also help point the way toward differential diagnoses.
“It’s one thing to say, ‘I think that child falls under the broad umbrella of ASD.’ But what if that umbrella was divided into subgroups — people who are on the spectrum for different reasons — that could be assigned the therapeutic approach that would best help them?” says O’Reilly, who also is a faculty affiliate in the university’s Artificial Intelligence Institute.
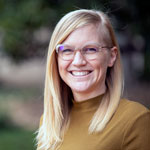
"The goal is to take all of our heart rate and visual attention data in very young infants and try to understand, using machine learning, how these features together might predict autism."
O’Reilly’s AI and machine learning tools aim to better understand and differentiate the physiological mechanisms involved in ASD using ECG (heart), EEG (brain) and other physiological and behavioral data of infants and children collected by Bradshaw’s, Hudac’s and other research groups at USC.
“The goal is to take all of our heart rate and visual attention data in very young infants and try to understand, using machine learning, how these features together might predict autism,” says Bradshaw, whose collaboration with O’Reilly is supported by a grant from the National Institutes of Health. “We call it modeling the autonomic, attentional and behavioral dynamics of autism.”
Bradshaw’s team is collecting data from 200 infants in South Carolina and beyond who have siblings already diagnosed with ASD, some with risk factors such as pre-term birth and others who are at a low likelihood of ASD with no known risk factors. The five-year project will follow the infants up to age three when they will receive a comprehensive autism diagnostic evaluation.
Hudac brought a large dataset of recorded brain activity to USC, a legacy of her nearly 15 years of research in exploring the biological process associated with autism and understanding how autistic individuals navigate the world. Her team continues to augment that dataset with new recordings.
“We’re trying to understand how individuals with ASD take in information, make sense of it and then choose to act in certain ways,” Hudac says. “There are key behavioral steps we can look at to see how different children have different brain processes for those steps. We’re recording every millisecond of their brain activity as they process and react to information, so we’re able to decode what’s happening and compare it with the brain activity of neurotypical children.”
Along with identifying biomarkers of ASD, Hudac says ASD research at USC and beyond could help inform new directions in ASD therapy.
“I think that that's everyone's goal. But as we start to navigate how different tools can get us there faster, there are still a lot of human limitations with imaging and EEG data that we have to process,” Hudac says. “It is more involved, trying to make sure that the data we get is going to help us in that therapeutic sense. I think it’s going to be absolutely pivotal, though, and no one field can make that happen alone — it's going to be an exciting decade in ASD research.”