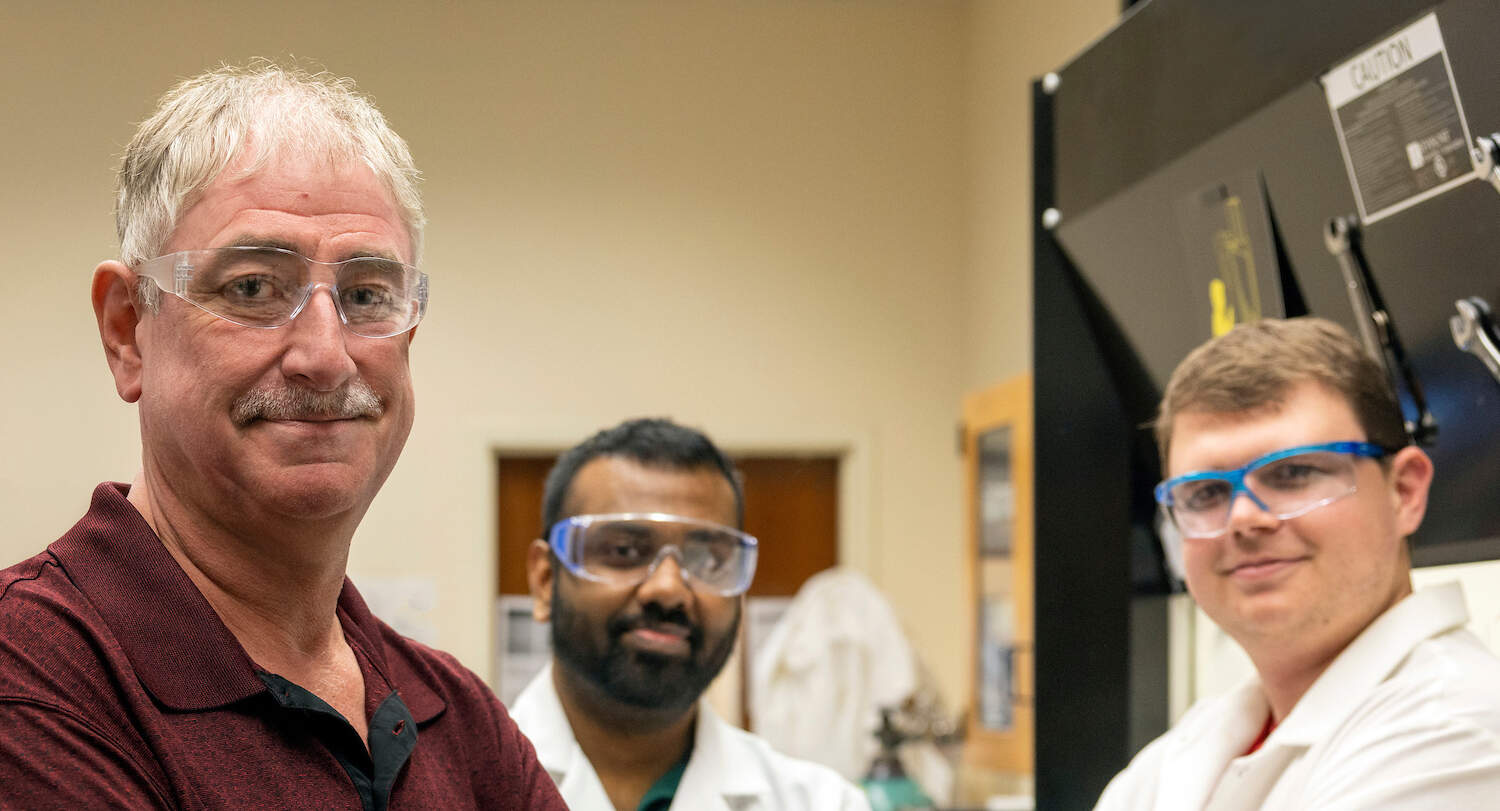
A leader in energy research, the University of South Carolina is part of the SC Nexus consortium, a regional technology and innovation hub for advanced energy research.
If Jochen Lauterbach’s research sounds like it borrows material from a sci-fi novel, there’s good reason. Artificial intelligence is the driver behind much of the work his research team does in its quest to forge new energy solutions.
Lauterbach, chair of USC’s SmartState Center for Strategic Approaches to the Generation of Electricity, is among a vanguard of scientists worldwide who are focused on producing carbon-free energy from nitrogen, hydrogen and the recycling of carbon from CO2. His work has implications not only for energy production, but also for the production of ammonia, a key base material for fertilizer, and therefore crucial to the global food supply.
The goals are cool, but the execution is complex.
“To do the things we’re trying to do, you have to react molecules with each other that don't like to react with each other,” says Lauterbach, whose research is funded by the Department of Energy and the Department of Defense. “The catalyst is the matchmaker between the different molecules, enabling reactions that otherwise wouldn't happen. We rely on AI-powered machine learning to create the optimal catalyst for a particular reaction.”
Lauterbach’s team designs novel catalysts at the atomic level, incorporating as many as 10 or more different components. Experiments are expensive and time consuming, which means the research crew needs to have a good idea where to look for the proverbial needle in the haystack when they’re searching for the best combination of catalyst materials.
“AI is very helpful because it's a very complex parameter space that we're in. AI helps us to accelerate the search process, save time and money and think outside of the box,” Lauterbach says. “And, because it involves machine learning, the AI system improves its knowledge over time and the predictions usually get better.”
For one project, Lauterbach’s team started with nine different formulations for a particular catalyst. After feeding those into the AI software, the computer suggested that they make 20 additional catalysts with specific molecular configurations. “We ended up with a catalyst that beat every other published catalyst out there for that particular reaction.”
The beauty of AI in experimental research, Lauterbach says, is that, unlike humans, the computer is open to any possible solution. “As scientists, we have a ‘chemical gut feeling,’ but we also have preconceived notions,” he says. “If we tell AI to find the best material for a catalyst, it doesn’t care if we say, ‘This is never going to work.’ It basically takes us to corners of the compositional space where we might not go as scientists.”
That happened on a Department of Energy-funded project when Lauterbach’s team, in conjunction with collaborators at the University of Buffalo, found catalyst materials for ammonia production that no one had previously tried — and the results were off-the-charts good.
“Ammonia production is one of the most important chemical reactions on the planet because fertilizer sustains so much of the world’s population,” Lauterbach says. “The current process for making ammonia is very energy intensive and runs at very high temperatures and high pressures — so it’s very energy intensive. If we can find new, cheaper catalysts that can run this reaction under milder conditions, we’ll save massive amounts of energy and improve safety. And AI is helping us find catalyst formulations that no one has ever published along those lines.
Lauterbach is hoping for similar results in a current U.S. Navy-funded project that’s aimed at extracting hydrogen from seawater to make ammonia, which in liquid form becomes nearly as energy-dense as kerosene or diesel fuel.
Lauterbach’s team has been using AI for the past 10 years to enhance its research efforts, one of the few experimentalist research groups in the U.S. to do so. Engineering colleges, including USC’s College of Engineering and Computing, are offering AI classes as part of their curriculum, “and we need to do this because we need to train the current generation of engineer students in this technology. They need to know this,” Lauterbach says. “I have grad students who have a job offer a year before they even graduate because they have a background in AI.”